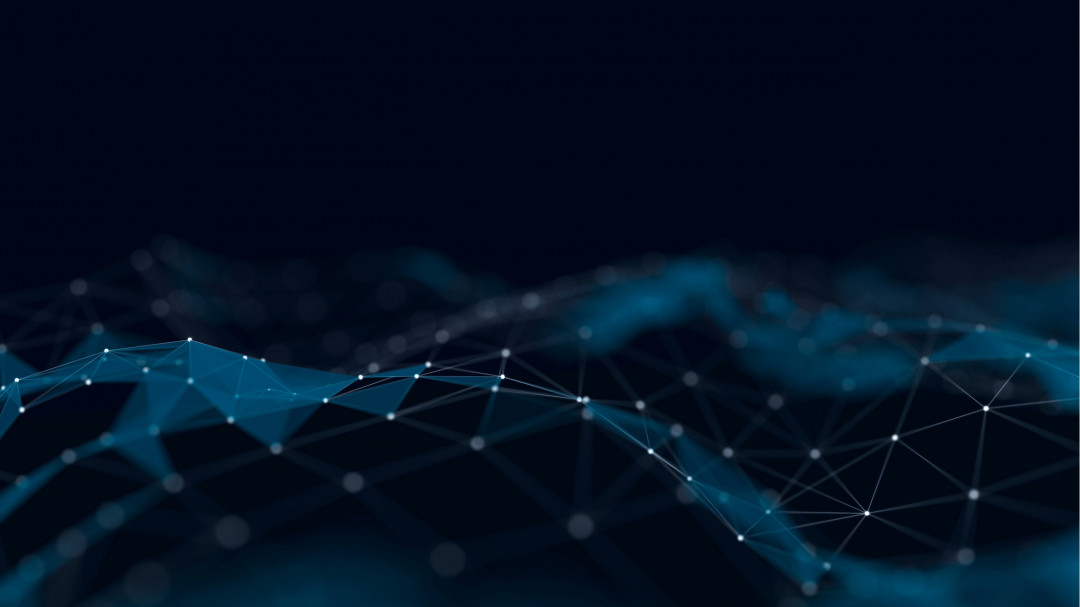
The crystalline microstructures of metallic materials are decisive for their properties. They have a significant influence on strength and deformation behaviour. So-called shape memory alloys, for example, change their shape due to temperature-related changes in the internal crystal structure. "Creating a suitable microstructure in these special materials is a major technical challenge. Checking this in detail using X-ray analyses is particularly complex," explains Prof. Dr Thomas Niendorf, Head of the Department of Metallic Materials.
Researchers often use X-ray diffraction methods for this purpose, in which a detector receives the diffracted X-rays and software visualises their intensity in a so-called pole figure. The material sample must be rotated and tilted until a pole figure is created from the measurement data. This can be used to calculate the arrangement and orientation of the crystals in the metal. These series of measurements often take several days. "With our specially developed algorithm, we are three times faster," reports David Meier, information scientist at the Helmholtz Centre Berlin and the Department of Intelligent Embedded Systems at the University of Kassel. The algorithm was trained using machine learning in such a way that it creates a complete reconstruction of the pole figure from just a small section of the real measurement data of a few hours, which differs only minimally from the original.
The scientists create pole figures of random arrangements of grains in the metal using a simulation. Using these simulated images, a customised deep learning architecture learns to generate the complete pole figure from a section. This "reconstruction network" can reconstruct the remaining areas for a small section of a real measured pole figure. The comparison of reconstruction and real, complete measurement results of the sample shows: The reconstruction network can analyse the sample with sufficient accuracy for the applied example. However, in order to statistically prove that the developed method works in other real scenarios, it must be evaluated in follow-up studies with other samples made of different materials. However, it seems certain that the combination of modern measurement technology and artificial intelligence will support the research and development of high-performance and durable materials in the future. The project results were published in the journal: Scientific Reports: 13, 5410 (2023): doi.org/10.1038/s41598-023-31580-1.